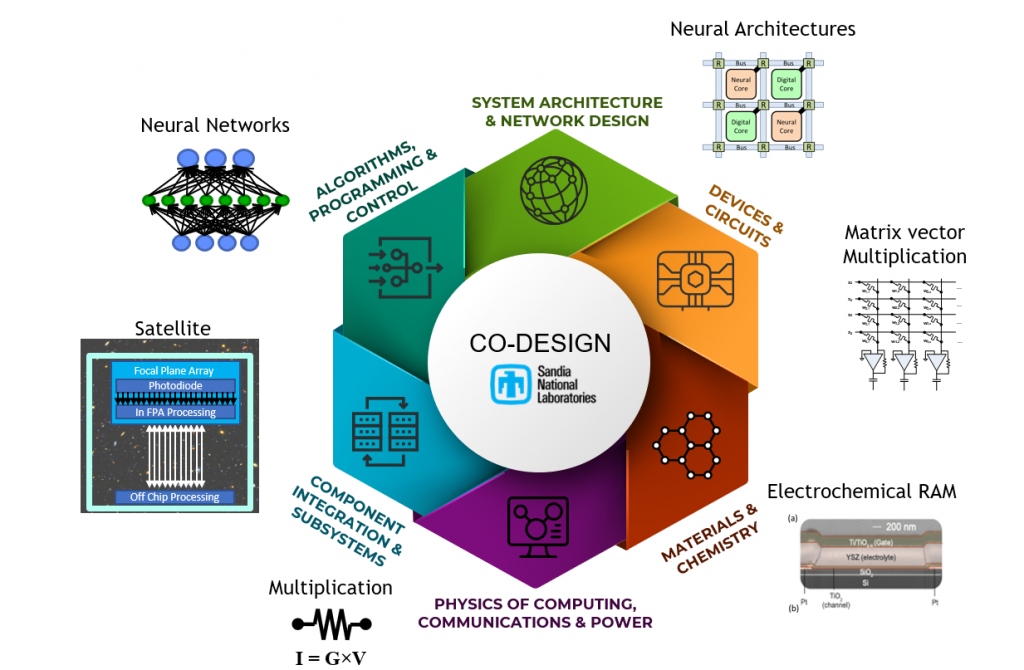
Novel material and device concepts previously took years for iteration. Discoveries in this LDRD project will now allow them to be iterated on in weeks thanks to a new easy fabrication substrate platform for novel devices. The team designed and taped out a microelectronics discovery platform or a “lab-on-a-chip” that has high-speed pulsing and high-resolution sensing that permits researchers to gauge fundamental limits of atomic switching in novel microelectronic devices including ECRAM and ReRAM. Additionally, the team released an updated microelectronics co-design software, CrossSim 2.0, a GPU-accelerated, Python-based crossbar simulator that allows researchers to take experimental data from the discovery platform and immediately model system-level performance for sensor processing mission applications. A strong relationship with National/Regional partner Arizona State University and a partnership with former Sandian, Matt Marinella, is providing a talent pipeline to multiple U.S. citizen students working on microelectronics co-design.
Sandia researchers linked to work
- Sapan Agarwal
- William Wahby
- Mieko Hirabayashi
- Patrick Finnegan
- Nad Gilbert
- Matthew Marinella
Sponsored by
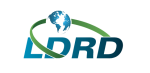
Associated Publications
Xiao, T. P., Feinberg, B., Bennett, C. H., Agrawal, V., Saxena, P., Prabhakar, V., … Marinella, M. J. (2022). An accurate, error-tolerant, and energy-efficient neural network inference engine based on SONOS analog memory. IEEE Transactions on Circuits and Systems. I, Regular Papers: A Publication of the IEEE Circuits and Systems Society, 69(4), 1480–1493. doi:10.1109/tcsi.2021.3134313
Agrawal, V., Xiao, T. P., Bennett, C. H., Feinberg, B., Shetty, S., Ramkumar, K., … Agarwal, S. (2022, December 3). Subthreshold operation of SONOS analog memory to enable accurate low-power neural network inference. 2022 International Electron Devices Meeting (IEDM). Presented at the 2022 IEEE International Electron Devices Meeting (IEDM), San Francisco, CA, USA. doi:10.1109/iedm45625.2022.10019564
Xiao, T. P., Feinberg, B., Bennett, C. H., Prabhakar, V., Saxena, P., Agrawal, V., … Marinella, M. J. (2022). On the accuracy of analog neural network inference accelerators [feature]. IEEE Circuits and Systems Magazine, 22(4), 26–48. doi:10.1109/mcas.2022.3214409
Keywords:
June 7, 2023