Timothy Michael Wildey
Scientific Machine Learning
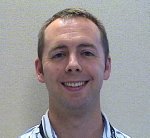
Scientific Machine Learning
(505) 844-0760
Sandia National Laboratories, New Mexico
P.O. Box 5800
Albuquerque, NM 87185-1318
Biography
Tim joined Sandia National Labs in January, 2011 following a postdoctoral fellowship at the University of Texas at Austin. His research interests are finite element and finite volume methods, discontinuous Galerkin methods, hybridized discretizations, a posteriori error analysis and estimation, uncertainty quantification, adjoint methods, multiphysics and multiscale problems, operator splitting and decomposition, computational fluid dynamics, shock-hydrodynamics, geomechanics, flow and transport in porous media, numerical linear algebra, domain decomposition, multilevel and multiscale preconditioners, and parallel computing.
Education
- Postdoctoral The University of Texas at Austin, Institute for Computational Engineering and Sciences Aug. 2007 – Dec. 2010
- Ph.D. Colorado State University, Mathematics Aug. 2007
- B.S. Michigan State University, Mathematics, May 2001
Publications
-
Eldred, M., Adams, B., Geraci, G., Portone, T., Ridgway, E., Stephens, J., Wildey, T., & Wildey, T. (2022). Deployment of Multifidelity Uncertainty Quantification for Thermal Battery Assessment Part I: Algorithms and Single Cell Results. https://doi.org/10.2172/1885882 Publication ID: 80135
-
Tran, A., Sun, J., Liu, D., Wang, Y., Wildey, T., & Wildey, T. (2022). A Stochastic Reduced-Order Model for Statistical Microstructure Descriptors Evolution. Journal of Computing and Information Science in Engineering, 22(6). https://doi.org/10.1115/1.4054237 Publication ID: 80523
-
Eldred, M., Geraci, G., Gorodetsky, A., Jakeman, J., Portone, T., Wildey, T., Rushdi, A.A., Seidl, D., & Seidl, D. (2021). The Dakota Project: Connecting the Pipeline from Uncertainty Quantification R&D to Mission Impact [Presentation]. https://www.osti.gov/biblio/1891078 Publication ID: 76127
-
Wildey, T., Butler, T., Jakeman, J., Tran, A., & Tran, A. (2021). Solving Stochastic Inverse Problems for Property-Structure Relationships in Computational Materials Science [Conference Presenation]. https://doi.org/10.2172/1890916 Publication ID: 79535
-
Wildey, T., Butler, T., Jakeman, J., & Jakeman, J. (2021). Combining Measure Theory and Bayes? Rule to Solve a Stochastic Inverse Problem [Conference Presenation]. https://doi.org/10.2172/1877851 Publication ID: 78143
-
Tran, A., Wildey, T., & Wildey, T. (2021). Solving inverse problems for process-structure linkages using asynchronous parallel Bayesian optimization [Conference Presenation]. https://doi.org/10.2172/1854075 Publication ID: 77439
-
Tran, A., Tranchida, J., Wildey, T., Thompson, A., & Thompson, A. (2021). Multi-fidelity ML/UQ and Bayesian Optimization for Materials Design: Application to Ternary Random Alloys [Conference Poster]. https://doi.org/10.2172/1853874 Publication ID: 77392
-
Tran, A., Wildey, T., & Wildey, T. (2021). Solving stochastic inverse problems for property-structure linkage using data-consistent inversion and ML [Conference Poster]. https://doi.org/10.2172/1848050 Publication ID: 77388
-
Tran, A., Wildey, T., Tranchida, J., Thompson, A., & Thompson, A. (2020). Multi-fidelity machine-learning with uncertainty quantification and Bayesian optimization for materials design: Application to ternary random alloys. Journal of Chemical Physics, 153(7). https://doi.org/10.1063/5.0015672 Publication ID: 73589
-
Tran, A., Wildey, T., Rodgers, T., & Rodgers, T. (2020). On supervised and unsupervised deep learning applications for materials informatics [Conference Poster]. https://www.osti.gov/biblio/1812467 Publication ID: 74399
-
Tran, A., Sun, J., Wang, Y., Liu, D., Wildey, T., & Wildey, T. (2020). Multiscale stochastic reduced-order model for uncertainty propagation using Fokker-Planck equation with microstructure evolution applications. arXiv preprint. https://www.osti.gov/biblio/1834331 Publication ID: 73191
-
Tran, A., Wildey, T., & Wildey, T. (2019). Materials informatics: data-driven materials design and uncertainty quantification perspectives [Conference Poster]. https://www.osti.gov/biblio/1643479 Publication ID: 66699
-
Wildey, T., Bruder, L., Bui-Thanh, T., Butler, T., Jakeman, J., Marvin, B., Tran, A., Walsh, S., & Walsh, S. (2019). Moving Beyond Forward Simulation to Enable Data-informed Physics-based Predictions [Presentation]. https://www.osti.gov/biblio/1646273 Publication ID: 66318
-
Wildey, T., Butler, T., Jakeman, J., & Jakeman, J. (2019). Convergence of Probability Densities using Approximate Models for Forward and Inverse Problems in Uncertainty Quantification [Conference Poster]. https://www.osti.gov/biblio/1641989 Publication ID: 64879
-
Tran, A., Wildey, T., McCann, S., & McCann, S. (2019). A sequential bi-fidelity constrained Bayesian optimization for design applications [Conference Poster]. https://www.osti.gov/biblio/1641565 Publication ID: 70358
-
Wildey, T., Butler, T., Jakeman, J., Bruder, L., & Bruder, L. (2019). Solving Stochastic Inverse Problems using Approximate Push-forward Densities based on a Multi-fidelity Monte Carlo Method [Conference Poster]. https://www.osti.gov/biblio/1641047 Publication ID: 69562
-
Wildey, T., Tran, A., & Tran, A. (2019). Using High Performance Computing to Enable Data-informed Multiscale Modeling with Applications to Additive Materials [Conference Poster]. https://www.osti.gov/biblio/1640837 Publication ID: 69247
-
Tran, A., Wang, Y., Wildey, T., & Wildey, T. (2019). A step towards a versatile Bayesian optimization: constrained asynchronous batch-parallel multi-fidelity and mixed-integer extensions [Conference Poster]. https://www.osti.gov/biblio/1640079 Publication ID: 68628
-
Wildey, T. (2019). Exploiting Low-dimensional Structure to Efficiently Perform Stochastic Inference for Prediction [Conference Poster]. https://www.osti.gov/biblio/1601938 Publication ID: 67079
-
Wildey, T., Butler, T., Jakeman, J., Bui-Thanh, T., Marvin, B., Bruder, L., & Bruder, L. (2019). Developing Scalable and Multi-fidelity Approaches for Push-forward Based Inference [Conference Poster]. https://www.osti.gov/biblio/1596420 Publication ID: 64514
-
Wildey, T., Marvin, B., Bui-Thanh, T., & Bui-Thanh, T. (2018). Scalable Approximations for the Consistent Bayes Method [Conference Poster]. https://www.osti.gov/biblio/1594647 Publication ID: 59327
-
Wildey, T., Butler, T., Jakeman, J., & Jakeman, J. (2018). The Consistent Bayesian Approach for Stochastic Inverse Problems [Conference Poster]. https://www.osti.gov/biblio/1592669 Publication ID: 59876
-
Wildey, T. (2018). Overview of Forward and Inverse Uncertainty Quantification Methods [Conference Poster]. https://www.osti.gov/biblio/1581941 Publication ID: 63733
-
Wildey, T., Butler, T., Jakeman, J., Marvin, B., & Marvin, B. (2018). Consistent Bayesian Inference with Push-forward Measures: Scalable Implementations and Applications [Conference Poster]. https://www.osti.gov/biblio/1567819 Publication ID: 62972
-
Jakeman, J., Butler, T., Eldred, M., Geraci, G., Gorodetsky, A., Wildey, T., & Wildey, T. (2018). Adaptive multi-index collocation for quantifying uncertainty [Conference Poster]. https://www.osti.gov/biblio/1806541 Publication ID: 63211
-
Wildey, T., Butler, T., Jakeman, J., Seidl, D., van Bloemen Waanders, B., & van Bloemen Waanders, B. (2018). Data-informed Multiscale Modeling of Additive Materials [Conference Poster]. https://www.osti.gov/biblio/1523778 Publication ID: 62297
-
Wildey, T., Butler, T., Jakeman, J., Walsh, S., & Walsh, S. (2018). Optimal Experimental Design for Prediction Using a Consistent Bayesian Approach [Conference Poster]. https://www.osti.gov/biblio/1507835 Publication ID: 61612
-
Seidl, D., van Bloemen Waanders, B., Wildey, T., & Wildey, T. (2018). Multiscale Interfaces for Large Scale Optimization [Conference Poster]. https://www.osti.gov/biblio/1525680 Publication ID: 61583
-
Walsh, S.N., Wildey, T., Jakeman, J., & Jakeman, J. (2018). Optimal experimental design using a consistent Bayesian approach. ASCE-ASME Journal of Risk and Uncertainty in Engineering Systems, Part B: Mechanical Engineering, 4(1). https://doi.org/10.1115/1.4037457 Publication ID: 56168
-
Roach, R.A., Jared, B.H., Cook, A., Keicher, D., van Bloemen Waanders, B., Swiler, L., Seidl, D., Wildey, T., Whetten, S., & Whetten, S. (2018). Born Qualified EAB Telecon [Presentation]. https://www.osti.gov/biblio/1514821 Publication ID: 60282
-
Wildey, T., Butler, T., Jakeman, J., & Jakeman, J. (2017). A Consistent Bayesian Approach for Solving Stochastic Inverse Problems [Conference Poster]. https://www.osti.gov/biblio/1469097 Publication ID: 58335
-
Wildey, T., Jakeman, J., Butler, T., & Butler, T. (2017). Advancing Beyond Interpretive Simulation to Inference for Prediction [Conference Poster]. https://www.osti.gov/biblio/1467988 Publication ID: 58203
-
Wildey, T., van Bloemen Waanders, B., Seidl, D., & Seidl, D. (2017). Adaptive Multiscale Modeling Using Generalized Mortar Methods [Conference Poster]. https://www.osti.gov/biblio/1513505 Publication ID: 57313
-
Mayr, M., Cyr, E., Shadid, J.N., Pawlowski, R., Wildey, T., Scovazzi, G., Zeng, X., Phillips, E., Conde, S., & Conde, S. (2017). Implicit-Explicit (IMEX) Time Integration for CFD & Multi-Physics Problems [Presentation]. https://www.osti.gov/biblio/1458227 Publication ID: 56716
-
Mayr, M., Cyr, E., Shadid, J.N., Pawlowski, R., Wildey, T., Scovazzi, G., Zeng, X., Phillips, E., Conde, S., & Conde, S. (2017). Implicit-Explicit (IMEX) Time Integration for Multi-Physics: Application to ALE-based CFD Simulations [Conference Poster]. https://www.osti.gov/biblio/1458228 Publication ID: 56717
-
Wildey, T., van Bloemen Waanders, B., Seidl, D., & Seidl, D. (2017). Multiscale Modeling Using Mortar Methods [Conference Poster]. https://www.osti.gov/biblio/1458195 Publication ID: 56724
-
Seidl, D., van Bloemen Waanders, B., Wildey, T., & Wildey, T. (2017). Multiscale Interfaces for Large Scale Optimization [Conference Poster]. https://www.osti.gov/biblio/1456522 Publication ID: 55715
-
van Bloemen Waanders, B., Wildey, T., Seidl, D., Li, H., & Li, H. (2017). Multiscale optimization under uncertainty for additive manufacturing [Conference Poster]. https://www.osti.gov/biblio/1426379 Publication ID: 55236
-
Wildey, T., Jakeman, J., Butler, T., & Butler, T. (2017). Efficient Sampling Strategies for the Consistent Bayesian Approach for Solving Stochastic Inverse Problems [Conference Poster]. https://www.osti.gov/biblio/1425298 Publication ID: 55046
-
van Bloemen Waanders, B., Wildey, T., Seidl, D., Li, H., & Li, H. (2017). Multiscale optimization under uncertainty [Presentation]. https://www.osti.gov/biblio/1458249 Publication ID: 55002
-
Seidl, D., van Bloemen Waanders, B., Wildey, T., & Wildey, T. (2017). Simultaneous Estimation of Material Parameters and Neumann Boundary Conditions in a Linear Elastic Model by PDE-Constrained Optimization [Conference Poster]. https://www.osti.gov/biblio/1458297 Publication ID: 54920
-
Wildey, T., Shadid, J.N., Cyr, E., Chaudry, J., & Chaudry, J. (2016). Adjoint Enhanced Methods for Uncertainty Quantification of Multiphysics Applications [Conference Poster]. https://www.osti.gov/biblio/1400047 Publication ID: 47138
-
Cyr, E., Shadid, J.N., Wildey, T., Phillips, E., Robinson, A., Miller, S., Pawlowski, R., & Pawlowski, R. (2016). Implicit-Explicit (IMEX) Time Integration for Multi-Physics: Application to ALE and Plasma Simulation [Conference Poster]. https://www.osti.gov/biblio/1401944 Publication ID: 47222
-
Wildey, T., Shadid, J.N., Cyr, E., & Cyr, E. (2016). Adjoint Enhanced Methods for Uncertainty Quantification of Multiphysics Applications [Conference Poster]. https://www.osti.gov/biblio/1367627 Publication ID: 50793
-
Wildey, T., Jakeman, J., Butler, T., & Butler, T. (2016). A Consistent Bayesian Approach for Stochastic Inverse Problems [Conference Poster]. https://www.osti.gov/biblio/1368940 Publication ID: 50347
-
Wildey, T., van Bloemen Waanders, B., Seidl, D., & Seidl, D. (2016). Uncertainty Quantification for Multiscale Mortar Methods [Conference Poster]. https://www.osti.gov/biblio/1368791 Publication ID: 50172
-
Carnes, B., Bova, S., Fisher, T., Weirs, V.G., Wildey, T., & Wildey, T. (2016). Discretization error transport for unstructured CFD [Conference Poster]. https://www.osti.gov/biblio/1422082 Publication ID: 50012
-
Wildey, T., van Bloemen Waanders, B., Seidl, D., Arbogast, T., Ganis, B., Girault, V., Pencheva, G., Wheeler, M., Xue, G., Yotov, I., Tavener, S., Vohralik, M., & Vohralik, M. (2016). Multiscale Mortar Methods: Theory Applications and Future Directions [Conference Poster]. https://www.osti.gov/biblio/1365248 Publication ID: 49573
-
van Bloemen Waanders, B., Wildey, T., Seidl, D., Li, H., & Li, H. (2016). Multiscale Optimization Under Uncertainty [Conference Poster]. https://www.osti.gov/biblio/1348106 Publication ID: 48990
-
Wildey, T., Cyr, E., Shadid, J.N., van Bloemen Waanders, B., Kouri, D.P., Bishop, J., Tavener, S., Butler, T., Prudhomme, S., Dawson, C., & Dawson, C. (2016). Utilizing Adjoint-Based Techniques to Improve the Accuracy and Reliability in Uncertainty Quantification [Conference Poster]. https://www.osti.gov/biblio/1345103 Publication ID: 48514
-
Wildey, T., Jakeman, J., & Jakeman, J. (2015). Adaptive Bayesian Inference for Prediction. https://doi.org/10.2172/1221574 Publication ID: 45595
-
Wildey, T., Shadid, J.N., Cyr, E., Jakeman, J., Butler, T., & Butler, T. (2015). Adjoint-Based a Posteriori Error Estimation and Uncertainty Quantification for Transient Nonlinear Problems with Discontinuous Solutions [Conference Poster]. https://www.osti.gov/biblio/1323036 Publication ID: 45375
-
Phipps, E., Red-Horse, J., Wildey, T., Constantine, P., Ghanem, R., Arnst, M., & Arnst, M. (2015). Stochastic Dimension Reduction of Multiphysics Systems through Measure Transformation [Conference Poster]. https://www.osti.gov/biblio/1321810 Publication ID: 45366
-
Wildey, T., Jakeman, J., Butler, T., Cyr, E., Shadid, J.N., & Shadid, J.N. (2015). Adjoint-Based a Posteriori Error Estimation and Uncertainty Quantification for Shock-Hydrodynamic Applications [Conference Poster]. https://www.osti.gov/biblio/1279685 Publication ID: 44737
-
Wildey, T., Jakeman, J., Butler, T., & Butler, T. (2015). Utilizing Adjoint-based Error Estimates to Adaptively Resolve Response Surface Approximations [Conference Poster]. https://www.osti.gov/biblio/1256570 Publication ID: 43836
-
Cyr, E., Shadid, J.N., Wildey, T., Hensinger, D., Robinson, A., Rider, W., Scovazzi, G., & Scovazzi, G. (2015). IMEX Lagrangian Methods [Conference Poster]. https://www.osti.gov/biblio/1253301 Publication ID: 43417
-
Wildey, T., Shadid, J.N., Cyr, E., Constantine, P., & Constantine, P. (2015). Enabling Efficient Uncertainty Quantification Using Adjoint-based Techniques. https://doi.org/10.2172/1179153 Publication ID: 43275
-
Fisher, T., Bova, S., Weirs, V.G., Wildey, T., & Wildey, T. (2015). Propagating Discretization Error Estimates in Compressible Turbulent Flow Simulations [Presentation]. https://www.osti.gov/biblio/1246879 Publication ID: 42861
-
Wildey, T., Cyr, E., Shadid, J.N., & Shadid, J.N. (2015). Utilizing Adjoint-Based Techniques to Effectively Perform UQ on Discontinuous Responses [Conference Poster]. https://www.osti.gov/biblio/1246277 Publication ID: 42478
-
Rider, W., Love, E., Wildey, T., & Wildey, T. (2014). Replacing Discon.nuous Functions in Limiters with Smooth Ones [Presentation]. https://www.osti.gov/biblio/1241667 Publication ID: 38694
-
Adams, B., Jakeman, J., Swiler, L., Stephens, J., Vigil, D., Wildey, T., Bauman, L., Bohnhoff, W., Dalbey, K., Eddy, J., Ebeida, M., Eldred, M., Hough, P., Hu, K., & Hu, K. (2014). Dakota, a multilevel parallel object-oriented framework for design optimization, parameter estimation, uncertainty quantification, and sensitivity analysis :. https://doi.org/10.2172/1177077 Publication ID: 41017
-
Adams, B., Jakeman, J., Swiler, L., Stephens, J., Vigil, D., Wildey, T., Bauman, L., Bohnhoff, W., Dalbey, K., Eddy, J., Ebeida, M., Eldred, M., Hough, P., Hu, K., & Hu, K. (2014). Dakota, a multilevel parallel object-oriented framework for design optimization, parameter estimation, uncertainty quantification, and sensitivity analysis version 6.0 theory manual. https://doi.org/10.2172/1177048 Publication ID: 40814
-
Eldred, M., Wildey, T., Dowding, K.J., & Dowding, K.J. (2014). Advanced UQ/QMU Methods for Abnormal Thermal Safety Studies [Presentation]. https://www.osti.gov/biblio/1692314 Publication ID: 40175
-
Wildey, T. (2014). Error decomposition and adaptivity for response surface approximations from PDEs with parametric uncertainty. SIAM/ASA Journal on Uncertainty Quantification. https://www.osti.gov/biblio/1141195 Publication ID: 40117
-
Swiler, L., Wildey, T., & Wildey, T. (2014). Sensitivity of precipitation to parameter values in the community atmosphere model version 5. https://doi.org/10.2172/1204103 Publication ID: 37049
-
Shadid, J.N., Pawlowski, R., Cyr, E., Wildey, T., & Wildey, T. (2014). Thermal hydraulic simulations, error estimation and parameter sensitivity studies in Drekar::CFD. https://doi.org/10.2172/1204072 Publication ID: 36835
-
Eldred, M., Jakeman, J., Wildey, T., & Wildey, T. (2013). Deployment of Scalable UQ Methods for High-Fidelity Simulation-based Applications within the DOE [Presentation]. https://www.osti.gov/biblio/1673675 Publication ID: 36511
-
Shadid, J.N., Pawlowski, R., Cyr, E., Wildey, T., Weber, P., & Weber, P. (2013). Drekar::CFD-A Trilinos Component-Based Software Flow Solver [Conference]. https://www.osti.gov/biblio/1296709 Publication ID: 36095
-
Wildey, T., Shadid, J.N., Cyr, E., & Cyr, E. (2013). Adjoint-Based Error Analysis for Stabilized Formulations and IMEX Schemes [Conference]. https://www.osti.gov/biblio/1140447 Publication ID: 36063
-
Jakeman, J., Wildey, T., & Wildey, T. (2013). Scalable Uncertainty Quantification Methods [Presentation]. https://www.osti.gov/biblio/1666168 Publication ID: 34628
-
Shadid, J.N., Wildey, T., Pawlowski, R., & Pawlowski, R. (2013). Error Estimation with Adjoints in Drekar: Applications to Fluid Flow and Magnetohydrodynamics Models [Presentation]. https://www.osti.gov/biblio/1661289 Publication ID: 33631
-
Red-Horse, J., Wildey, T., & Wildey, T. (2013). Stochastic Dimension Reduction of Multiphysics Systems through Measure Transformation [Conference]. https://www.osti.gov/biblio/1145287 Publication ID: 32256
-
Jakeman, J., Wildey, T., & Wildey, T. (2013). Quantifying Uncertainty using a-posteriori Enhanced Sparse Grid Approximations [Conference]. https://www.osti.gov/biblio/1063316 Publication ID: 32169
-
Phipps, E., Wildey, T., & Wildey, T. (2013). Efficient uncertainty propagation for network multiphysics systems. Proposed for publication in International Journal for Numerical Methods in Engineering.. https://www.osti.gov/biblio/1063360 Publication ID: 31415
-
Wildey, T., Swiler, L., & Swiler, L. (2012). Progress on Calibration of 2-Degree Simulations from CAM5 [Presentation]. https://www.osti.gov/biblio/1648264 Publication ID: 30744
-
Wildey, T. (2012). Error Control for Output QoIs Under Uncertainty [Conference]. https://www.osti.gov/biblio/1062303 Publication ID: 30554
-
Wildey, T. (2012). Error Decomposition and Adaptive Refinement for Stochastic Differential Equations [Presentation]. https://www.osti.gov/biblio/1658506 Publication ID: 29331
-
Jakeman, J., Wildey, T., Eldred, M., & Eldred, M. (2012). Adaptive sparse grids for uncertainty quantication Enhancing approximations using a posteriori error estimation [Conference]. https://www.osti.gov/biblio/1073416 Publication ID: 29105
-
Eldred, M., Wildey, T., & Wildey, T. (2012). Propagation of model form uncertainty for thermal hydraulics using RANS turbulence models in Drekar. https://doi.org/10.2172/1051699 Publication ID: 29125
-
Wildey, T., Shadid, J.N., & Shadid, J.N. (2012). Goal-oriented a posteriori analysis of stabilized finite element methods [Conference]. https://www.osti.gov/biblio/1073289 Publication ID: 29275
-
Wildey, T. (2012). PROPAGATION OF UNCERTAINTIES USING IMPROVED SURROGATE MODELS. Proposed for publication in SIAM Journal on Uncertainty Quantification.. https://www.osti.gov/biblio/1064108 Publication ID: 28851
-
Wildey, T. (2012). A posteriori Analysis of Interior Penalty Discontinuous Galerkin Methods. Proposed for publication in SIAM Journal of Numerical Analysis.. https://www.osti.gov/biblio/1067830 Publication ID: 28017
-
Red-Horse, J., Wildey, T., & Wildey, T. (2012). Stochastic Dimension Reduction Techniques for Uncertainty Quantification of Multiphysics Systems [Conference]. https://www.osti.gov/biblio/1117584 Publication ID: 27536
-
Pawlowski, R., Shadid, J.N., Wildey, T., & Wildey, T. (2011). Embedded UQ and QoI/Adjoints in Drekar: New Directions [Conference]. https://www.osti.gov/biblio/1113265 Publication ID: 25656
-
Wildey, T. (2011). Quantification and Reduction of Uncertainties for Regional Scale Climate Predictions [Presentation]. https://www.osti.gov/biblio/1662132 Publication ID: 24184
-
Wildey, T. (2011). Preconditioning for Mixed Finite Element Formulations of Elliptic Problems [Conference]. https://www.osti.gov/biblio/1106970 Publication ID: 22828
-
Wildey, T. (2011). Multiscale Mortar Methods for Flow and Mechanics in Porous Media [Conference]. https://www.osti.gov/biblio/1288934 Publication ID: 22560
-
Wildey, T. (2011). A Dirichlet-to-Neumann (DtN) multigrid algorithm for locally conservative methods [Conference]. https://www.osti.gov/biblio/1288632 Publication ID: 22028