Craig M. Vineyard
Computer Scientist
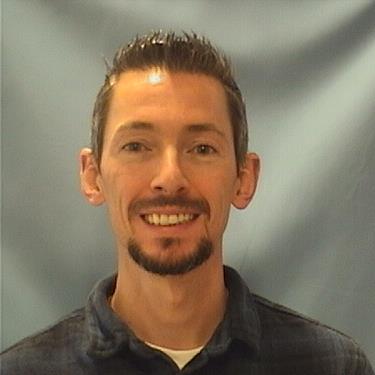
Computer Scientist
Biography
Craig M. Vineyard, PhD has degrees in computer engineering with expertise in machine learning and neuromorphic computing. He has been at Sandia National Laboratories for over 15 years pursuing computing research and development for national security. This includes performing foundational research on how advanced computing technologies can impact a range of applications from scientific computing to remote sensing. His research contributions include machine learning algorithm analysis and development; neuromorphic computing algorithms and architectures; computer architecture analysis, simulation, benchmarking; game theory; and information theoretic analysis.
Education
Ph.D., Computer Engineering, University of New Mexico 2015
M.S., Computer Engineering, University of New Mexico 2008
B.S., Computer Engineering, University of New Mexico 2006
Publications
- Dick, Robert P., Rob Aitken, Jace Mogill, John Paul Strachan, Kirk Bresniker, Wei Lu, Yorie Nakahira, Zhiyong li, Matthew J. Marinella, William Severa, A. Alec Talin, Craig M. Vineyard, Suhas Kumar, Christian Mailhiot, and Lennie Klebanoff. ”Research Challenges for Energy-Efficient Computing in Automated Vehicles.” Computer 56, no. 3 (2023): 47-58.
- Melzer, R., Severa, W. M., & Vineyard, C. M. (2022, May). Exploring SAR ATR with neural networks: going beyond accuracy. In Automatic Target Recognition XXXII (Vol. 12096, pp. 125-144). SPIE.
- Vineyard, Craig, Suma Cardwell, Frances Chance, Srideep Musuvathy, Fred Rothganger, William Severa, John Smith, Corinne Teeter, Felix Wang, and James Aimone. “Neural Mini-Apps as a Tool for Neuromorphic Computing Insight.” In Neuro-Inspired Computational Elements Conference, pp. 40-49. 2022.
- L´eonard, F., Fuller, E. J., Teeter, C. M., & Vineyard, C. M. (2022). High accuracy single-layer free-space diffractive neuromorphic classifiers for spatially incoherent light. Optics Express, 30(8), 12510-12520.
- J.B. Aimone, A.J. Hill, W.M. Severa, C. M. Vineyard “Spiking Neural Streaming Binary Arithmetic” in n 2021 International Conference on Rebooting Computing (ICRC) (pp. 79-83). IEEE.
- F. Leonard, A. Backer, E. Fuller, C. Teeter, C. M. Vineyard “Co-Design of Free-Space Metasurface Optical Neuromorphic Classifiers for High Performance” in ACS Photonics 2021
- R. Melzer, W.M. Severa, M. Plagge, C. M. Vineyard, “Exploring Characteristics of Neural Network Architectures for Enabling SAR ATR,” in Automatic Target Recognition XXXI. (Vol. 11729, p. 1172909) International Society for Optics and Photonics
- S.G. Cardwell, C. M. Vineyard, W.M. Severa, F.S. Chance, F. Rothganger, F. Wang, S. Musuvathy, C. Teeter, and J.B. Aimone, “Truly Heterogeneous HPC: Co-design to Achieve What Science Needs from HPC,” in Smoky Mountains Computational Science and Engineering Conference pp. 349-365
- C. M. Vineyard, S. Green, and M. Plagge, “Comparing neural accelerators & neuromorphic the false idol of operations,” in Proceedings of the 8th Annual Neuro-inspired Computational Elements Workshop, 2020.
- W. M. Severa, R. Dellana, and C. M. Vineyard, “Effective Pruning of Binary Activation Neural Networks,” in Proceedings of the International Conference on Neuromorphic Systems, pp. 1–5, 2020.
- H. Dbouk, H. Geng, C. M. Vineyard, and N. R. Shanbhag, “Low-complexity fixed-point convolutional neural networks for automatic target recognition,” in Proceedings of the International Conference on Acoustics, Speech, and Signal Processing, 2020.
- S. Sanyal, A. Ankit, C. M. Vineyard, and K. Roy, “Energy-Efficient Target Recognition using ReRAM Crossbars for Enabling On-Device Intelligence,” in Proceedings of IEEE Workshop on Signal Processing Systems (SiPS), 2020
- S. Green, C. M. Vineyard, R. Helinski, and C. K. Ko¸c, “Rapdarts: Resourceaware progressive differentiable architecture search,” Proceedings of the International Joint Conference on Neural Networks (IJCNN), 2020.
- F. Chance, J. B. Aimone, S. M. Musuvathy, M. R. Smith, C. M. Vineyard, and F. Wang, “Crossing the cleft: Communication challenges between neuroscience and artificial intelligence,” Frontiers in Computational Neuroscience 2020.
- C. M. Vineyard, S. Green, W. M. Severa, and C¸ . K. Ko¸c, “Benchmarking eventdriven neuromorphic architectures,” in Proceedings of the International Conference on Neuromorphic Systems, pp. 1–5, 2019.
- J. B. Aimone, W. Severa, and C. M. Vineyard, “Composing neural algorithms with fugu,” in Proceedings of the International Conference on Neuromorphic Systems, pp. 1–8, 2019.
- C. M. Vineyard, W. Severa, M. Kagie, A. Scholand, and P. Hays, “A resurgence
in neuromorphic architectures enabling remote sensing computation,” in 2019
IEEE Space Computing Conference (SCC), pp. 33–40, IEEE, 2019. - W. Severa, A. Hill, C. M. Vineyard, M. Kagie, R. Dellana, L. Reeder, F. Wang,
J. Aimone, and Yanguas-Gil, “A comprehensive approach to building a neuromorphic
platform for remote computation,” DoD Journal of Research & Engineering, vol. 2,
no. 2, pp. 59–67, 2019 (microelectronics special edition) (August). - C. M. Vineyard, R. Dellana, J. B. Aimone, F. Rothganger, and W. M. Severa,
“Low-power deep learning inference using the spinnaker neuromorphic platform,”
in Proceedings of the 7th Annual Neuro-inspired Computational Elements Workshop,
pp. 1–7, 2019. - S. Green, C. M. Vineyard, and C. K. Ko¸c, “Distillation strategies for proximal policy optimization,” arXiv preprint arXiv:1901.08128, 2019.
- W. Severa, A. J. Hill, C. M. Vineyard, R. Dellana, L. Reeder, F. Wang, J. B. Aimone, and A. Yanguas-Gil, “Building a comprehensive neuromorphic platform for remote computation,” in Gomactech, 2019.
- W. Severa, C. M. Vineyard, R. Dellana, S. J. Verzi, and J. B. Aimone, “Training deep neural networks for binary communication with the whetstone method,” Nature Machine Intelligence, vol. 1, no. 2, pp. 86–94, 2019.
- S. J. Verzi, C. M. Vineyard, and J. B. Aimone, “Neural-inspired anomaly detection,” in International Conference on Complex Systems, pp. 202–209, Springer, 2018.
- S. J. Verzi, F. Rothganger, O. D. Parekh, T.-T. Quach, N. E. Miner, C. M. Vineyard, C. D. James, and J. B. Aimone, “Computing with spikes: The advantage of fine-grained timing,” Neural computation, vol. 30, no. 10, pp. 2660–2690, 2018.
- S. Green, C. M. Vineyard, and C. K. Ko¸c, “Impacts of mathematical optimizations on reinforcement learning policy performance,” in 2018 International Joint Conference
on Neural Networks (IJCNN), pp. 1–8, IEEE, 2018. - A. J. Hill, J. W. Donaldson, F. H. Rothganger, C. M. Vineyard, D. R. Follett, P. L. Follett, M. R. Smith, S. J. Verzi, W. Severa, F. Wang, et al., “A spike-timing neuromorphic architecture,” in 2017 IEEE International Conference on Rebooting Computing (ICRC), pp. 1–8, IEEE, 2017.
- M. R. Smith, A. J. Hill, K. D. Carlson, C. M. Vineyard, J. Donaldson, D. R. Follett, P. L. Follett, J. H. Naegle, C. D. James, and J. B. Aimone, “A novel digital neuromorphic architecture efficiently facilitating complex synaptic response functions applied to liquid state machines,” in 2017 International Joint Conference on Neural Networks (IJCNN), pp. 2421–2428, IEEE, 2017.
- T. J. Draelos, N. E. Miner, C. C. Lamb, J. A. Cox, C. M. Vineyard, K. D. Carlson, W. M. Severa, C. D. James, and J. B. Aimone, “Neurogenesis deep learning: Extending deep networks to accommodate new classes,” in 2017 International Joint Conference on Neural Networks (IJCNN), pp. 526–533, IEEE, 2017.
- S. J. Verzi, C. M. Vineyard, E. D. Vugrin, M. Galiardi, C. D. James, and J. B. Aimone, “Optimization-based computation with spiking neurons,” in 2017 International Joint Conference on Neural Networks (IJCNN), pp. 2015–2022, IEEE, 2017.
- C. D. James, J. B. Aimone, N. E. Miner, C. M. Vineyard, F. H. Rothganger, K. D. Carlson, S. A. Mulder, T. J. Draelos, A. Faust, M. J. Marinella, et al., “A historical survey of algorithms and hardware architectures for neural-inspired and neuromorphic computing applications,” Biologically Inspired Cognitive Architectures, vol. 19, pp. 49–64, 2017.
- C. M. Vineyard and S. J. Verzi, “Overcoming the static learning bottleneck-the need for adaptive neural learning,” in 2016 IEEE International Conference on Rebooting Computing (ICRC), pp. 1–3, IEEE, 2016.
- C. M. Vineyard, S. J. Verzi, C. D. James, and J. B. Aimone, “Quantifying neural information content: A case study of the impact of hippocampal adult neurogenesis,” in 2016 International Joint Conference on Neural Networks (IJCNN), pp. 5181–5188, IEEE, 2016.
- C. M. Vineyard, S. J. Verzi, C. D. James, J. B. Aimone, and G. L. Heileman, “Mapreduce svm game,” Procedia Computer Science, vol. 53, no. C, 2015.
- C. M. Vineyard, S. J. Verzi, C. D. James, J. B. Aimone, and G. L. Heileman, “Repeated play of the svm game as a means of adaptive classification,” in 2015 International Joint Conference on Neural Networks (IJCNN), pp. 1–8, IEEE, 2015.
- C. M. Vineyard, S. J. Verzi, T. P. Caudell, M. L. Bernard, and J. B. Aimone, “Adult neurogenesis: Implications on human and computational decision making,” in International Conference on Augmented Cognition, pp. 531–540, Springer, 2013.
- C. M. Vineyard, G. R. Emmanuel, S. J. Verzi, and G. L. Heileman, “A game theoretic model of neurocomputation.,” in BICA, pp. 373–374, Springer, 2012.
- C. M. Vineyard, J. B. Aimone, and G. R. Emmanuel, “Neurogenesis in a high resolution dentate gyrus model,” in Biologically Inspired Cognitive Architectures 2012, pp. 371–372, Springer, 2013.
- C. M. Vineyard, S. J. Verzi, M. L. Bernard, S. E. Taylor, I. Dubicka, and T. P. Caudell, “A multi-modal network architecture for knowledge discovery,” Security Informatics, vol. 1, no. 1, pp. 1–12, 2012.
- C. M. Vineyard, G. L. Heileman, S. J. Verzi, and R. Jordan, “Game theoretic mechanism design applied to machine learning classification,” in 2012 3rd International Workshop on Cognitive Information Processing (CIP), pp. 1–5, IEEE, 2012.
- C. M. Vineyard, K. Lakkaraju, J. Collard, and S. J. Verzi, “The impact of attitude resolve on population wide attitude change,” in International Conference on Social Computing, Behavioral-Cultural Modeling, and Prediction, pp. 322–330, Springer, 2012.
- C. M. Vineyard, S. J. Verzi, M. L. Bernard, and T. P. Caudell, “A multimodal hypertensor architecture for association formation.,” in BICA, pp. 419–424, 2011.
- C. M. Vineyard, S. J. Verzi, M. L. Bernard, S. E. Taylor, W. L. Shaneyfelt, I. Dubicka, J. T. McClain, and T. P. Caudell, “A neurophysiologically inspired hippocampus based associative-art artificial neural network architecture,” in The 2011 International Joint Conference on Neural Networks, pp. 2100–2105, IEEE, 2011.
- C. M. Vineyard, M. L. Bernard, S. E. Taylor, T. P. Caudell, P. D. Watson, S. J. Verzi, N. J. Cohen, and H. Eichenbaum, “A neurologically plausible artificial neural network computational architecture of episodic memory and recall.,” in BICA, pp. 175–180, 2010.
- C. M. Vineyard, S. E. Taylor, M. L. Bernard, S. J. Verzi, T. P. Caudell, G. L. Heileman, and P. Watson, “A cortical-hippocampal neural architecture for episodic memory with information theoretic model analysis,” in World MultiConference on Systemics, Cybernetics and Informatics, pp. 281–285, 2010.
- C. Vineyard, S. Taylor, M. Bernard, S. Verzi, J. Morrow, P. Watson, H. Eichenbaum, M. Healy, T. Caudell, and N. Cohen, “Episodic memory modeled by an integrated cortical-hippocampal neural architecture,” in Human Behavior and Computational Modeling Conference, pp. 23–24, 2009.
- S. E. Taylor, M. L. Bernard, S. J. Verzi, J. D. Morrow, C. M. Vineyard, M. J. Healy, and T. P. Caudell, “Temporal semantics: An adaptive resonance theory approach,” in 2009 International Joint Conference on Neural Networks, pp. 3111–3117, IEEE, 2009.
- S. E. Taylor, C. M. Vineyard, M. J. Healy, T. P. Caudell, N. J. Cohen, P. Watson, S. J. Verzi, J. D. Morrow, M. L. Bernard, and H. Eichenbaum, “Memory in silico: Building a neuromimetic episodic cognitive model,” in 2009 WRI World Congress on Computer Science and Information Engineering, vol. 5, pp. 733–737, IEEE, 2009.
Chapters
- S. Green, C. M. Vineyard, and C¸ . K. Ko¸c, “Mathematical optimizations for deep learning,” in Cyber-Physical Systems Security, pp. 69–92, Springer, 2018.
Miscellaneous Publications
- 2019 Sandia National Laboratories High Performance Computing Annual Report. “Neural Exploration & Research Lab” Pg. 23-24
- https://sandia.gov/news/publications/computing reports/index.html
- 2020 Sandia National Laboratories High Performance Computing Annual Report. “Evaluate. Innovate. Repeat. Computing Teams at Sandia Chart New Course for Next-generation Architecture” Pg. 58-62
- https://www.sandia.gov/news/publications/computing reports/ assets/documents/
2020 HPC AnnualReport.pdf
- https://www.sandia.gov/news/publications/computing reports/ assets/documents/
- 2022 Sandia National Laboratories High Performance Computing Annual Report
- “Neural Mini-Apps: Lighter, Faster Diagnostic Tests for Neuromorphic Computing”
Pg. 14-15 - “Neuromorphic Object Recognition at the Speed of Light” Pg. 16-19
https://www.sandia.gov/news/publications/hpc-annual-reports/report/2022-hpcreport/
- “Neural Mini-Apps: Lighter, Faster Diagnostic Tests for Neuromorphic Computing”
- Computer Science Research Institute (CSRI) Summer Proceedings 2020. “Evolving
Spiking Circuit Motifs Using Weight Agnostic Neural networks” Pg. 3-10 SAND2020-
12580R - SAND Report. “Neural Inspired Computation Remote Sensing Platform” SAND2019-
11291 - SAND Report. “An introduction to neuromorphic computing and its potential
impact for unattended ground sensors” SAND2021-13025R - SAND Report. “SEEK: Scoping neuromorphic architecture impact enabling advanced
sensing capabilities” SAND2022-14058 - SAND Report. “Full Stack Neuromorphic Technologies and Capabilities” SAND2022-
10373M https://ip.sandia.gov/opportunity/full-stack-neuromorphic/ - Green, Sam, Craig M. Vineyard, and Cetin Kaya Ko¸c. ”Distillation Strategies for
Proximal Policy Optimization.” arXiv preprint arXiv:1901.08128 (2019). - “NeuroBench: Advancing Neuromorphic Computing through Collaborative, Fair
and Representative Benchmarking” arXiv:2304.04650
https://neurobench.ai/
Patents & Trademarks
Issued
- Patent 10303697 “Temporal Data System”
- Patent 11436475 “Anomaly Detection with Spiking Neural Networks”
Filed
- Optimization Computation with Spiking Neurons (Application 15/837,326)
- Devices and Methods for Increasing the Speed or Power Efficiency of a Computer
- When Performing Machine Learning Using Spiking Neural Circuits (Application
- 16/013,810)
- System and Method for Training Deep Artificial Neural Networks (Application
- 16/146,904)
- Increasing Classifier Robustness via Binary Activation Neural Networks (SD 15133)
- Algorithmic Architecture Co-Design and Exploration (Application 17/461,847)
- Sequence-Based Anomaly Detection with Hierarchical Spiking Neural Networks (Application
- 17/890,843)